Your cart is currently empty!
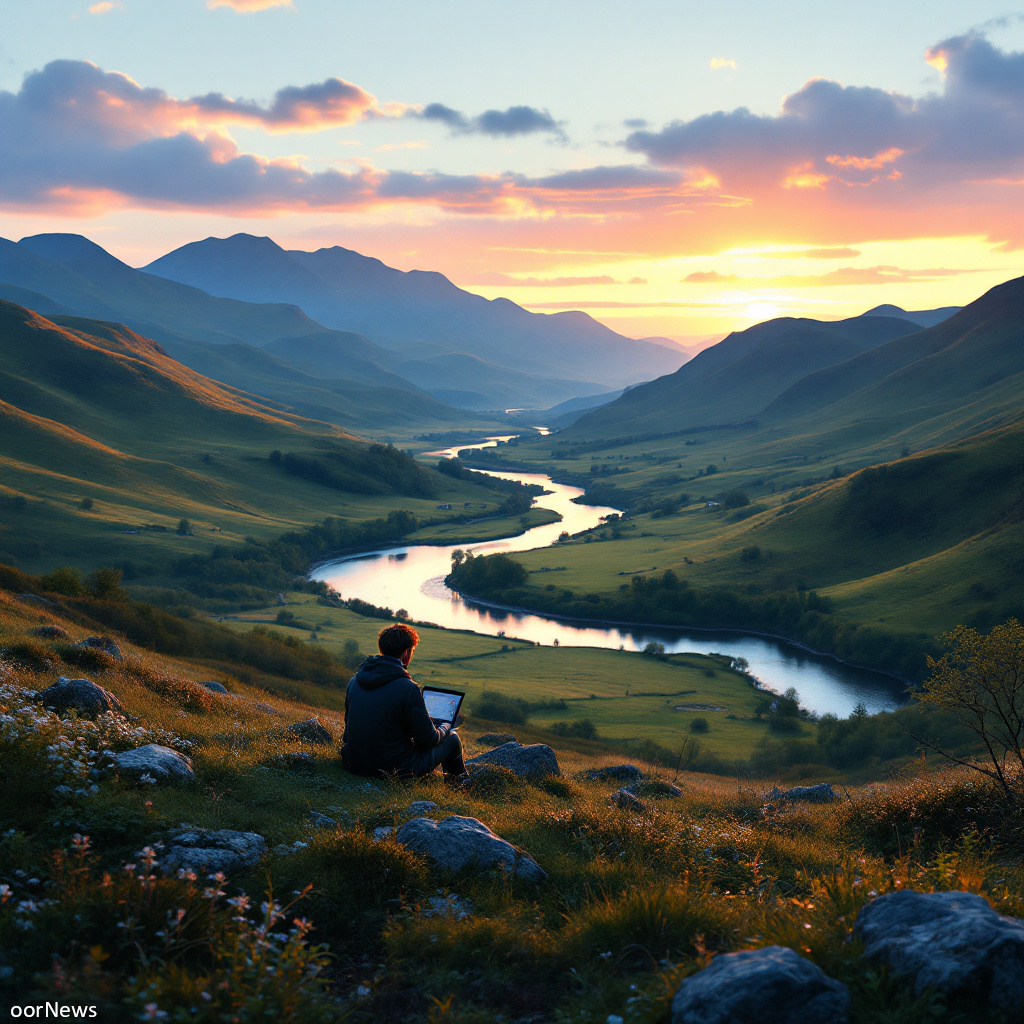
New Software Model Makes Geospatial Data Analysis Greener
A team of scientists from the University of Glasgow has unveiled a groundbreaking software model that promises to make the analysis of geospatial data more eco-friendly. The model, named ‘GeoAggregator’, uses machine learning to lessen the computing power needed to handle vast and intricate geospatial datasets.
As GPS and satellite data become more common, the world gathers massive amounts of geospatial information each day. Yet, making sense of this data is no small feat. Traditional methods and even some AI models struggle to grasp the complex spatial ties within the data. GeoAggregator tackles these hurdles with a lightweight AI model that deftly examines how nearby places affect each other and how patterns shift from one spot to another. It is faster, more scalable, and demands fewer resources than its predecessors, opening doors for researchers and policymakers alike.
One standout feature of GeoAggregator is its Gaussian-biased local attention mechanism. This allows the model to zero in on nearby data points while keeping the bigger picture in mind. Such an approach boosts predictions for various spatial data issues, from forecasting air pollution to spotting housing price trends and studying poverty spread.
The model’s Cartesian attention mechanism keeps it light while ensuring high accuracy. Even as datasets grow, GeoAggregator processes them with ease, a feat many traditional AI models cannot match. Tests show that GeoAggregator performs as well as or better than other leading methods, using far fewer resources. This makes it a practical choice for real-world tasks where speed and efficiency are key.
The researchers have shared their code as open-source, urging others to use and refine it. As interest in location-based data swells, tools like GeoAggregator can turn complex geospatial information into meaningful insights about how people and places interact.
Dr. Mingshu Wang from the University of Glasgow’s School of Geographical & Earth Sciences, a co-author of the study, highlights the model’s potential: “GeoAggregator marks a big leap in making complex data analysis more efficient and accessible. We did all our data analysis on a single laptop, showing just how effective this tool is.”
The team is working on an open-source Python package to make the model freely available. This effort is part of ongoing PhD research by Rui Deng, who will continue to enhance the model over the next two years. Dr. Ziqi Li, an Assistant Professor at Florida State University, is also a co-author of the paper.
This research is part of the University of Glasgow’s growing expertise in AI and machine learning, following the launch of their Centre for Data Science & AI. The School of Geographical & Earth Sciences has recently received funding to lead the Exascale computing for Earth, Environmental, and Sustainability Solutions consortium, which will train new PhD students to develop software for environmental applications on next-gen computing systems. The team’s paper will be presented at the AAAI Conference on Artificial Intelligence this week.